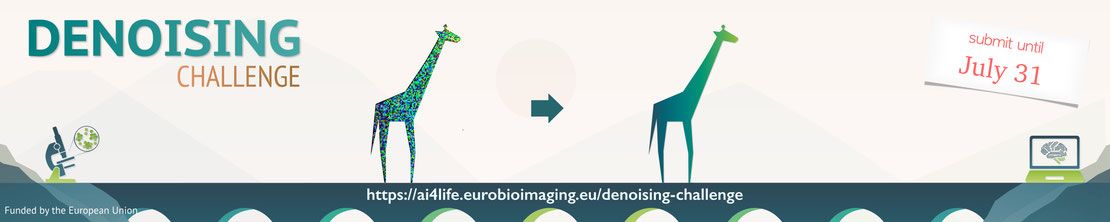
Hagen dataset¶
In [1]:
Copied!
# Imports necessary to execute the code
from pathlib import Path
import matplotlib.pyplot as plt
import numpy as np
import pooch
import tifffile
from PIL import Image
from careamics import CAREamist
from careamics.config import create_n2v_configuration
from careamics.utils import autocorrelation
# use n2v2
use_n2v2 = False
# folder in which to save all the data
root = Path("hagen")
# Imports necessary to execute the code from pathlib import Path import matplotlib.pyplot as plt import numpy as np import pooch import tifffile from PIL import Image from careamics import CAREamist from careamics.config import create_n2v_configuration from careamics.utils import autocorrelation # use n2v2 use_n2v2 = False # folder in which to save all the data root = Path("hagen")
Import the dataset¶
In [ ]:
Copied!
# download the data using pooch
data_root = root / "data"
dataset_url = "https://zenodo.org/records/10925855/files/noisy.tiff?download=1"
file = pooch.retrieve(
url=dataset_url,
known_hash="ff12ee5566f443d58976757c037ecef8bf53a00794fa822fe7bcd0dd776a9c0f",
path=data_root,
)
# download the data using pooch data_root = root / "data" dataset_url = "https://zenodo.org/records/10925855/files/noisy.tiff?download=1" file = pooch.retrieve( url=dataset_url, known_hash="ff12ee5566f443d58976757c037ecef8bf53a00794fa822fe7bcd0dd776a9c0f", path=data_root, )
Data description¶
In [3]:
Copied!
data_description = (
"Laser scanning fluorescence microscopy imaging of actin in bovine pulmonary "
"artery endothelial cells, acquired with a low signal-to-noise ratio. This dataset "
"is a subset of Dataset 4, as described in the paper by Hagen, G.M. et al., 2021 "
"(Fluorescence Microscopy Datasets for Training Deep Neural Networks, "
"GigaScience). The data is available on Zenodo at "
"https://zenodo.org/records/10925855."
)
print(data_description)
data_description = ( "Laser scanning fluorescence microscopy imaging of actin in bovine pulmonary " "artery endothelial cells, acquired with a low signal-to-noise ratio. This dataset " "is a subset of Dataset 4, as described in the paper by Hagen, G.M. et al., 2021 " "(Fluorescence Microscopy Datasets for Training Deep Neural Networks, " "GigaScience). The data is available on Zenodo at " "https://zenodo.org/records/10925855." ) print(data_description)
Laser scanning fluorescence microscopy imaging of actin in bovine pulmonary artery endothelial cells, acquired with a low signal-to-noise ratio. This dataset is a subset of Dataset 4, as described in the paper by Hagen, G.M. et al., 2021 (Fluorescence Microscopy Datasets for Training Deep Neural Networks, GigaScience). The data is available on Zenodo at https://zenodo.org/records/10925855.
Visualize data¶
In [4]:
Copied!
# load training and validation image and show them side by side
train_image = tifffile.imread(file)
print(f"Image shape: {train_image.shape}")
fig, ax = plt.subplots(1, 3, figsize=(15, 5))
ax[0].imshow(
train_image[0],
cmap="gray",
vmin=np.percentile(train_image[0], 1),
vmax=np.percentile(train_image[0], 99),
)
ax[0].set_title("Image 1")
ax[1].imshow(
train_image[1],
cmap="gray",
vmin=np.percentile(train_image[1], 1),
vmax=np.percentile(train_image[1], 99),
)
ax[1].set_title("Image 2")
ax[2].imshow(
train_image[2],
cmap="gray",
vmin=np.percentile(train_image[2], 1),
vmax=np.percentile(train_image[2], 99),
)
ax[2].set_title("Image 3")
# load training and validation image and show them side by side train_image = tifffile.imread(file) print(f"Image shape: {train_image.shape}") fig, ax = plt.subplots(1, 3, figsize=(15, 5)) ax[0].imshow( train_image[0], cmap="gray", vmin=np.percentile(train_image[0], 1), vmax=np.percentile(train_image[0], 99), ) ax[0].set_title("Image 1") ax[1].imshow( train_image[1], cmap="gray", vmin=np.percentile(train_image[1], 1), vmax=np.percentile(train_image[1], 99), ) ax[1].set_title("Image 2") ax[2].imshow( train_image[2], cmap="gray", vmin=np.percentile(train_image[2], 1), vmax=np.percentile(train_image[2], 99), ) ax[2].set_title("Image 3")
Image shape: (79, 1024, 1024)
Out[4]:
Text(0.5, 1.0, 'Image 3')
Compute autocorrelation¶
In [5]:
Copied!
autocorr = autocorrelation(train_image[0])
# crop the correlation around (0, 0)
midpoint = train_image[0].shape[0] // 2
crop_size = 20
slices = (
slice(midpoint - crop_size, midpoint + crop_size),
slice(midpoint - crop_size, midpoint + crop_size),
)
# plot autocorrelation
fig, ax = plt.subplots(1, 2, figsize=(15, 5))
ax[0].imshow(train_image[0], cmap="gray")
ax[0].set_title("Image 1")
ax[1].imshow(autocorr[slices], cmap="gray")
ax[1].set_title("Autocorrelation")
autocorr = autocorrelation(train_image[0]) # crop the correlation around (0, 0) midpoint = train_image[0].shape[0] // 2 crop_size = 20 slices = ( slice(midpoint - crop_size, midpoint + crop_size), slice(midpoint - crop_size, midpoint + crop_size), ) # plot autocorrelation fig, ax = plt.subplots(1, 2, figsize=(15, 5)) ax[0].imshow(train_image[0], cmap="gray") ax[0].set_title("Image 1") ax[1].imshow(autocorr[slices], cmap="gray") ax[1].set_title("Autocorrelation")
Out[5]:
Text(0.5, 1.0, 'Autocorrelation')
In [6]:
Copied!
# create configuration
algo = "n2v2" if use_n2v2 else "n2v"
config = create_n2v_configuration(
experiment_name="hagen_" + algo,
data_type="array",
axes="SYX",
patch_size=(64, 64),
batch_size=32,
num_epochs=10,
use_n2v2=use_n2v2,
)
# change augmentations
config.data_config.transforms[0].flip_y = False # do not flip y
config.data_config.transforms.pop(1) # remove 90 degree rotations
print(config)
# create configuration algo = "n2v2" if use_n2v2 else "n2v" config = create_n2v_configuration( experiment_name="hagen_" + algo, data_type="array", axes="SYX", patch_size=(64, 64), batch_size=32, num_epochs=10, use_n2v2=use_n2v2, ) # change augmentations config.data_config.transforms[0].flip_y = False # do not flip y config.data_config.transforms.pop(1) # remove 90 degree rotations print(config)
{'algorithm_config': {'algorithm': 'n2v', 'loss': 'n2v', 'lr_scheduler': {'name': 'ReduceLROnPlateau', 'parameters': {}}, 'model': {'architecture': 'UNet', 'conv_dims': 2, 'depth': 2, 'final_activation': 'None', 'in_channels': 1, 'independent_channels': True, 'n2v2': False, 'num_channels_init': 32, 'num_classes': 1}, 'optimizer': {'name': 'Adam', 'parameters': {'lr': 0.0001}}}, 'data_config': {'axes': 'SYX', 'batch_size': 32, 'data_type': 'array', 'patch_size': [64, 64], 'transforms': [{'flip_x': True, 'flip_y': False, 'name': 'XYFlip', 'p': 0.5}, {'masked_pixel_percentage': 0.2, 'name': 'N2VManipulate', 'roi_size': 11, 'strategy': 'uniform', 'struct_mask_axis': 'none', 'struct_mask_span': 5}]}, 'experiment_name': 'hagen_n2v', 'training_config': {'accumulate_grad_batches': 1, 'check_val_every_n_epoch': 1, 'checkpoint_callback': {'auto_insert_metric_name': False, 'mode': 'min', 'monitor': 'val_loss', 'save_last': True, 'save_top_k': 3, 'save_weights_only': False, 'verbose': False}, 'enable_progress_bar': True, 'gradient_clip_algorithm': 'norm', 'max_steps': -1, 'num_epochs': 10, 'precision': '32'}, 'version': '0.1.0'}
Train¶
In [ ]:
Copied!
# instantiate a CAREamist
careamist = CAREamist(
source=config,
work_dir=root / algo,
)
# train
careamist.train(
train_source=train_image,
val_percentage=0.0,
val_minimum_split=10, # use 10 patches as validation
)
# instantiate a CAREamist careamist = CAREamist( source=config, work_dir=root / algo, ) # train careamist.train( train_source=train_image, val_percentage=0.0, val_minimum_split=10, # use 10 patches as validation )
Predict¶
In [ ]:
Copied!
prediction = careamist.predict(
source=train_image,
tile_size=(256, 256),
tile_overlap=(48, 48),
batch_size=1,
tta=False,
)
prediction = careamist.predict( source=train_image, tile_size=(256, 256), tile_overlap=(48, 48), batch_size=1, tta=False, )
Save predictions¶
In [9]:
Copied!
pred_folder = root / ("results_" + algo)
pred_folder.mkdir(exist_ok=True, parents=True)
final_data = np.concatenate(prediction)
tifffile.imwrite(pred_folder / "prediction.tiff", final_data)
pred_folder = root / ("results_" + algo) pred_folder.mkdir(exist_ok=True, parents=True) final_data = np.concatenate(prediction) tifffile.imwrite(pred_folder / "prediction.tiff", final_data)
Visualize the prediction¶
In [10]:
Copied!
n = 5
fig, ax = plt.subplots(n, 2, figsize=(10, n * 5))
for i in range(n):
ax[i, 0].imshow(
train_image[i],
cmap="gray",
vmin=np.percentile(train_image[i], 1),
vmax=np.percentile(train_image[i], 99),
)
ax[i, 0].set_title(f"Noisy - Image {i}")
ax[i, 1].imshow(
prediction[i].squeeze(),
cmap="gray",
vmin=np.percentile(prediction[i], 1),
vmax=np.percentile(prediction[i], 99),
)
ax[i, 1].set_title(f"Prediction - Prediction {i}")
n = 5 fig, ax = plt.subplots(n, 2, figsize=(10, n * 5)) for i in range(n): ax[i, 0].imshow( train_image[i], cmap="gray", vmin=np.percentile(train_image[i], 1), vmax=np.percentile(train_image[i], 99), ) ax[i, 0].set_title(f"Noisy - Image {i}") ax[i, 1].imshow( prediction[i].squeeze(), cmap="gray", vmin=np.percentile(prediction[i], 1), vmax=np.percentile(prediction[i], 99), ) ax[i, 1].set_title(f"Prediction - Prediction {i}")
Create cover¶
In [11]:
Copied!
# create a cover image
im_idx = 0
cv_image_noisy = train_image[im_idx]
cv_image_pred = prediction[im_idx].squeeze()
# create image
cover = np.zeros((256, 256))
(height, width) = cv_image_noisy.shape
assert height > 256
assert width > 256
# normalize train and prediction
norm_noise = (cv_image_noisy - cv_image_noisy.min()) / (cv_image_noisy.max() - cv_image_noisy.min())
norm_pred = (cv_image_pred - cv_image_pred.min()) / (cv_image_pred.max() - cv_image_pred.min())
# fill in halves
cover[:, :256 // 2] = norm_noise[height // 2 - 256 // 2:height // 2 + 256 // 2, width // 2 - 256 // 2:width // 2]
cover[:, 256 // 2:] = norm_pred[height // 2 - 256 // 2:height // 2 + 256 // 2, width // 2:width // 2 + 256 // 2]
# plot the single image
plt.imshow(cover, cmap="gray")
# save the image
im = Image.fromarray(cover * 255)
im = im.convert('L')
im.save("Hagen_N2V.jpeg")
# create a cover image im_idx = 0 cv_image_noisy = train_image[im_idx] cv_image_pred = prediction[im_idx].squeeze() # create image cover = np.zeros((256, 256)) (height, width) = cv_image_noisy.shape assert height > 256 assert width > 256 # normalize train and prediction norm_noise = (cv_image_noisy - cv_image_noisy.min()) / (cv_image_noisy.max() - cv_image_noisy.min()) norm_pred = (cv_image_pred - cv_image_pred.min()) / (cv_image_pred.max() - cv_image_pred.min()) # fill in halves cover[:, :256 // 2] = norm_noise[height // 2 - 256 // 2:height // 2 + 256 // 2, width // 2 - 256 // 2:width // 2] cover[:, 256 // 2:] = norm_pred[height // 2 - 256 // 2:height // 2 + 256 // 2, width // 2:width // 2 + 256 // 2] # plot the single image plt.imshow(cover, cmap="gray") # save the image im = Image.fromarray(cover * 255) im = im.convert('L') im.save("Hagen_N2V.jpeg")
In [12]:
Copied!
general_description = (
"This model is a UNet trained with the Noise2Void algorithm to denoise images. The "
"training data consists of laser scanning confocal microscopy images of actin in "
"bovine pulmonary artery endothelial cells. The notebook used to train this model "
"is available on the CAREamics documentation website at the following link: "
"https://careamics.github.io/0.1/applications/Noise2Void/Hagen/."
)
print(general_description)
general_description = ( "This model is a UNet trained with the Noise2Void algorithm to denoise images. The " "training data consists of laser scanning confocal microscopy images of actin in " "bovine pulmonary artery endothelial cells. The notebook used to train this model " "is available on the CAREamics documentation website at the following link: " "https://careamics.github.io/0.1/applications/Noise2Void/Hagen/." ) print(general_description)
This model is a UNet trained with the Noise2Void algorithm to denoise images. The training data consists of laser scanning confocal microscopy images of actin in bovine pulmonary artery endothelial cells. The notebook used to train this model is available on the CAREamics documentation website at the following link: https://careamics.github.io/0.1/applications/Noise2Void/Hagen/.
In [ ]:
Copied!
# Export the model
careamist.export_to_bmz(
path_to_archive="hagen_n2v_model.zip",
friendly_model_name="Hagen_N2V",
input_array=train_image[[im_idx], 384:640, 256:512].astype(np.float32),
authors=[{"name": "CAREamics authors", "affiliation": "Human Technopole"}],
general_description=general_description,
data_description=data_description,
)
# Export the model careamist.export_to_bmz( path_to_archive="hagen_n2v_model.zip", friendly_model_name="Hagen_N2V", input_array=train_image[[im_idx], 384:640, 256:512].astype(np.float32), authors=[{"name": "CAREamics authors", "affiliation": "Human Technopole"}], general_description=general_description, data_description=data_description, )