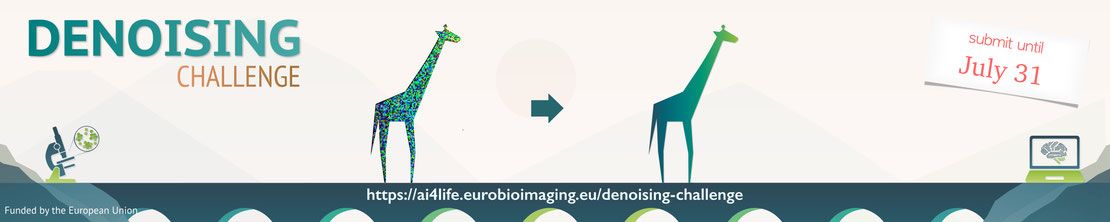
SUPPORT dataset¶
In [1]:
Copied!
# Imports necessary to execute the code
from pathlib import Path
import matplotlib.pyplot as plt
import pooch
import tifffile
import numpy as np
from PIL import Image
from careamics import CAREamist
from careamics.config import create_n2v_configuration
from careamics.utils import autocorrelation
# use n2v2
use_n2v2 = False
# folder in which to save all the data
root = Path("support")
# Imports necessary to execute the code from pathlib import Path import matplotlib.pyplot as plt import pooch import tifffile import numpy as np from PIL import Image from careamics import CAREamist from careamics.config import create_n2v_configuration from careamics.utils import autocorrelation # use n2v2 use_n2v2 = False # folder in which to save all the data root = Path("support")
Import the dataset¶
In [ ]:
Copied!
# download the data using pooch
data_root = root / "data"
dataset_url = "https://zenodo.org/records/10925939/files/noisy.tiff?download=1"
file = pooch.retrieve(
url=dataset_url,
known_hash="c09a0748a67a9364f257e0aff9502a283b8794f35381577f5dfea6ac1bd01e03",
path=data_root,
)
# download the data using pooch data_root = root / "data" dataset_url = "https://zenodo.org/records/10925939/files/noisy.tiff?download=1" file = pooch.retrieve( url=dataset_url, known_hash="c09a0748a67a9364f257e0aff9502a283b8794f35381577f5dfea6ac1bd01e03", path=data_root, )
Visualize data¶
In [3]:
Copied!
# load training and validation image and show them side by side
train_image = tifffile.imread(file)
print(f"Image shape: {train_image.shape}")
fig, ax = plt.subplots(1, 4, figsize=(20, 5))
ax[0].imshow(train_image[100])
ax[0].set_title("Slice 100")
ax[1].imshow(train_image[400])
ax[1].set_title("Slice 400")
ax[2].imshow(train_image[700])
ax[2].set_title("Slice 700")
ax[3].imshow(train_image[900])
ax[3].set_title("Slice 900")
# load training and validation image and show them side by side train_image = tifffile.imread(file) print(f"Image shape: {train_image.shape}") fig, ax = plt.subplots(1, 4, figsize=(20, 5)) ax[0].imshow(train_image[100]) ax[0].set_title("Slice 100") ax[1].imshow(train_image[400]) ax[1].set_title("Slice 400") ax[2].imshow(train_image[700]) ax[2].set_title("Slice 700") ax[3].imshow(train_image[900]) ax[3].set_title("Slice 900")
Image shape: (1001, 1024, 1024)
Out[3]:
Text(0.5, 1.0, 'Slice 900')
Compute autocorrelation¶
In [4]:
Copied!
autocorr = autocorrelation(train_image[400])
# crop the correlation around (0, 0)
midpoint = train_image[400].shape[0] // 2
crop_size = 20
slices = (
slice(midpoint - crop_size, midpoint + crop_size),
slice(midpoint - crop_size, midpoint + crop_size),
)
# plot autocorrelation
fig, ax = plt.subplots(1, 2, figsize=(15, 5))
ax[0].imshow(train_image[400])
ax[0].set_title("Image 1")
ax[1].imshow(autocorr[slices], cmap="gray")
ax[1].set_title("Autocorrelation")
autocorr = autocorrelation(train_image[400]) # crop the correlation around (0, 0) midpoint = train_image[400].shape[0] // 2 crop_size = 20 slices = ( slice(midpoint - crop_size, midpoint + crop_size), slice(midpoint - crop_size, midpoint + crop_size), ) # plot autocorrelation fig, ax = plt.subplots(1, 2, figsize=(15, 5)) ax[0].imshow(train_image[400]) ax[0].set_title("Image 1") ax[1].imshow(autocorr[slices], cmap="gray") ax[1].set_title("Autocorrelation")
Out[4]:
Text(0.5, 1.0, 'Autocorrelation')
In [5]:
Copied!
# create configuration
algo = "n2v2" if use_n2v2 else "n2v"
config = create_n2v_configuration(
experiment_name="support_" + algo,
data_type="array",
axes="ZYX",
patch_size=(16, 64, 64),
batch_size=2,
num_epochs=20,
use_n2v2=use_n2v2,
)
# change augmentations
config.data_config.transforms[0].flip_y = False # do not flip y
config.data_config.transforms.pop(1) # remove 90 degree rotations
print(config)
# create configuration algo = "n2v2" if use_n2v2 else "n2v" config = create_n2v_configuration( experiment_name="support_" + algo, data_type="array", axes="ZYX", patch_size=(16, 64, 64), batch_size=2, num_epochs=20, use_n2v2=use_n2v2, ) # change augmentations config.data_config.transforms[0].flip_y = False # do not flip y config.data_config.transforms.pop(1) # remove 90 degree rotations print(config)
{'algorithm_config': {'algorithm': 'n2v', 'loss': 'n2v', 'lr_scheduler': {'name': 'ReduceLROnPlateau', 'parameters': {}}, 'model': {'architecture': 'UNet', 'conv_dims': 3, 'depth': 2, 'final_activation': 'None', 'in_channels': 1, 'independent_channels': True, 'n2v2': False, 'num_channels_init': 32, 'num_classes': 1}, 'optimizer': {'name': 'Adam', 'parameters': {'lr': 0.0001}}}, 'data_config': {'axes': 'ZYX', 'batch_size': 2, 'data_type': 'array', 'patch_size': [16, 64, 64], 'transforms': [{'flip_x': True, 'flip_y': False, 'name': 'XYFlip', 'p': 0.5}, {'masked_pixel_percentage': 0.2, 'name': 'N2VManipulate', 'roi_size': 11, 'strategy': 'uniform', 'struct_mask_axis': 'none', 'struct_mask_span': 5}]}, 'experiment_name': 'support_n2v', 'training_config': {'checkpoint_callback': {'auto_insert_metric_name': False, 'mode': 'min', 'monitor': 'val_loss', 'save_last': True, 'save_top_k': 3, 'save_weights_only': False, 'verbose': False}, 'num_epochs': 20}, 'version': '0.1.0'}
Train¶
In [ ]:
Copied!
# instantiate a CAREamist
careamist = CAREamist(
source=config,
work_dir=root / algo,
)
# train
careamist.train(
train_source=train_image,
val_percentage=0.0,
val_minimum_split=10, # use 10 patches as validation
)
# instantiate a CAREamist careamist = CAREamist( source=config, work_dir=root / algo, ) # train careamist.train( train_source=train_image, val_percentage=0.0, val_minimum_split=10, # use 10 patches as validation )
Predict¶
In [ ]:
Copied!
prediction = careamist.predict(
source=train_image,
tile_size=(32, 128, 128),
tile_overlap=(8, 48, 48),
batch_size=1,
tta=False,
)
prediction = careamist.predict( source=train_image, tile_size=(32, 128, 128), tile_overlap=(8, 48, 48), batch_size=1, tta=False, )
Save predictions¶
In [8]:
Copied!
pred_folder = root / ("results_" + algo)
pred_folder.mkdir(exist_ok=True, parents=True)
tifffile.imwrite(pred_folder / "prediction.tiff", prediction[0])
pred_folder = root / ("results_" + algo) pred_folder.mkdir(exist_ok=True, parents=True) tifffile.imwrite(pred_folder / "prediction.tiff", prediction[0])
Visualize the prediction¶
In [9]:
Copied!
zs = [100, 250, 500, 750, 900]
fig, ax = plt.subplots(len(zs), 2, figsize=(10, 5 * len(zs)))
for i, z in enumerate(zs):
ax[i, 0].imshow(train_image[z])
ax[i, 0].set_title(f"Noisy - Plane {z}")
ax[i, 1].imshow(prediction[0].squeeze()[z])
ax[i, 1].set_title(f"Prediction - Plane {z}")
zs = [100, 250, 500, 750, 900] fig, ax = plt.subplots(len(zs), 2, figsize=(10, 5 * len(zs))) for i, z in enumerate(zs): ax[i, 0].imshow(train_image[z]) ax[i, 0].set_title(f"Noisy - Plane {z}") ax[i, 1].imshow(prediction[0].squeeze()[z]) ax[i, 1].set_title(f"Prediction - Plane {z}")
Create cover¶
In [10]:
Copied!
# create a cover image
im_idx = 500
cv_image_noisy = train_image[im_idx]
cv_image_pred = prediction[0].squeeze()[im_idx]
# create image
cover = np.zeros((256, 256))
(height, width) = cv_image_noisy.shape
assert height > 256
assert width > 256
# normalize train and prediction
norm_noise = (cv_image_noisy - cv_image_noisy.min()) / (cv_image_noisy.max() - cv_image_noisy.min())
norm_pred = (cv_image_pred - cv_image_pred.min()) / (cv_image_pred.max() - cv_image_pred.min())
# fill in halves
cover[:, :256 // 2] = norm_noise[height // 2 - 256 // 2:height // 2 + 256 // 2, width // 2 - 256 // 2:width // 2]
cover[:, 256 // 2:] = norm_pred[height // 2 - 256 // 2:height // 2 + 256 // 2, width // 2:width // 2 + 256 // 2]
# plot the single image
plt.imshow(cover, cmap="gray")
# save the image
im = Image.fromarray(cover * 255)
im = im.convert('L')
im.save("SUPPORT_N2V.jpeg")
# create a cover image im_idx = 500 cv_image_noisy = train_image[im_idx] cv_image_pred = prediction[0].squeeze()[im_idx] # create image cover = np.zeros((256, 256)) (height, width) = cv_image_noisy.shape assert height > 256 assert width > 256 # normalize train and prediction norm_noise = (cv_image_noisy - cv_image_noisy.min()) / (cv_image_noisy.max() - cv_image_noisy.min()) norm_pred = (cv_image_pred - cv_image_pred.min()) / (cv_image_pred.max() - cv_image_pred.min()) # fill in halves cover[:, :256 // 2] = norm_noise[height // 2 - 256 // 2:height // 2 + 256 // 2, width // 2 - 256 // 2:width // 2] cover[:, 256 // 2:] = norm_pred[height // 2 - 256 // 2:height // 2 + 256 // 2, width // 2:width // 2 + 256 // 2] # plot the single image plt.imshow(cover, cmap="gray") # save the image im = Image.fromarray(cover * 255) im = im.convert('L') im.save("SUPPORT_N2V.jpeg")