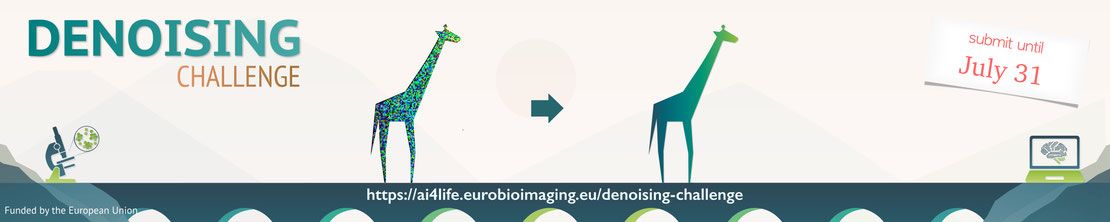
W2S dataset¶
In [1]:
Copied!
# Imports necessary to execute the code
from pathlib import Path
import matplotlib.pyplot as plt
import numpy as np
import pooch
import tifffile
from careamics import CAREamist
from careamics.config import create_n2v_configuration
# use n2v2
use_n2v2 = False
# folder in which to save all the data
root = Path("w2s")
# Imports necessary to execute the code from pathlib import Path import matplotlib.pyplot as plt import numpy as np import pooch import tifffile from careamics import CAREamist from careamics.config import create_n2v_configuration # use n2v2 use_n2v2 = False # folder in which to save all the data root = Path("w2s")
Import the dataset¶
In [ ]:
Copied!
# download the data using pooch
data_root = root / "data"
dataset_url = "https://zenodo.org/records/10925783/files/noisy.tiff?download=1"
file = pooch.retrieve(
url=dataset_url,
known_hash="b5cb1dbcb86ce72b8d6e0268498712974b9f38d2fc9e18e8a66673a34aa84215",
path=data_root,
)
# download the data using pooch data_root = root / "data" dataset_url = "https://zenodo.org/records/10925783/files/noisy.tiff?download=1" file = pooch.retrieve( url=dataset_url, known_hash="b5cb1dbcb86ce72b8d6e0268498712974b9f38d2fc9e18e8a66673a34aa84215", path=data_root, )
Visualize data¶
In [3]:
Copied!
# load training and validation image and show them side by side
train_image = tifffile.imread(file)
print(f"Image shape: {train_image.shape}")
fig, ax = plt.subplots(1, 3, figsize=(15, 5))
ax[0].imshow(train_image[0][0], cmap="gray")
ax[0].set_title("Channel 1")
ax[1].imshow(train_image[0][1], cmap="gray")
ax[1].set_title("Channel 2")
ax[2].imshow(train_image[0][2], cmap="gray")
ax[2].set_title("Channel 3")
# load training and validation image and show them side by side train_image = tifffile.imread(file) print(f"Image shape: {train_image.shape}") fig, ax = plt.subplots(1, 3, figsize=(15, 5)) ax[0].imshow(train_image[0][0], cmap="gray") ax[0].set_title("Channel 1") ax[1].imshow(train_image[0][1], cmap="gray") ax[1].set_title("Channel 2") ax[2].imshow(train_image[0][2], cmap="gray") ax[2].set_title("Channel 3")
Image shape: (120, 3, 512, 512)
Out[3]:
Text(0.5, 1.0, 'Channel 3')
In [4]:
Copied!
# create configuration
algo = "n2v2" if use_n2v2 else "n2v"
config = create_n2v_configuration(
experiment_name="w2s_" + algo,
data_type="array",
axes="SCYX",
patch_size=(64, 64),
batch_size=32,
num_epochs=15,
n_channels=3,
use_n2v2=use_n2v2,
)
print(config)
# create configuration algo = "n2v2" if use_n2v2 else "n2v" config = create_n2v_configuration( experiment_name="w2s_" + algo, data_type="array", axes="SCYX", patch_size=(64, 64), batch_size=32, num_epochs=15, n_channels=3, use_n2v2=use_n2v2, ) print(config)
{'algorithm_config': {'algorithm': 'n2v', 'loss': 'n2v', 'lr_scheduler': {'name': 'ReduceLROnPlateau', 'parameters': {}}, 'model': {'architecture': 'UNet', 'conv_dims': 2, 'depth': 2, 'final_activation': 'None', 'in_channels': 3, 'independent_channels': True, 'n2v2': False, 'num_channels_init': 32, 'num_classes': 3}, 'optimizer': {'name': 'Adam', 'parameters': {'lr': 0.0001}}}, 'data_config': {'axes': 'SCYX', 'batch_size': 32, 'data_type': 'array', 'patch_size': [64, 64], 'transforms': [{'flip_x': True, 'flip_y': True, 'name': 'XYFlip', 'p': 0.5}, {'name': 'XYRandomRotate90', 'p': 0.5}, {'masked_pixel_percentage': 0.2, 'name': 'N2VManipulate', 'roi_size': 11, 'strategy': 'uniform', 'struct_mask_axis': 'none', 'struct_mask_span': 5}]}, 'experiment_name': 'w2s_n2v', 'training_config': {'checkpoint_callback': {'auto_insert_metric_name': False, 'mode': 'min', 'monitor': 'val_loss', 'save_last': True, 'save_top_k': 3, 'save_weights_only': False, 'verbose': False}, 'num_epochs': 15}, 'version': '0.1.0'}
Train¶
In [ ]:
Copied!
# instantiate a CAREamist
careamist = CAREamist(
source=config,
work_dir=root / algo,
)
# train
careamist.train(
train_source=train_image,
val_percentage=0.0,
val_minimum_split=10, # use 10 patches as validation
)
# instantiate a CAREamist careamist = CAREamist( source=config, work_dir=root / algo, ) # train careamist.train( train_source=train_image, val_percentage=0.0, val_minimum_split=10, # use 10 patches as validation )
Predict¶
In [ ]:
Copied!
prediction = careamist.predict(
source=train_image,
tile_size=(256, 256),
tile_overlap=(48, 48),
batch_size=1,
)
prediction = careamist.predict( source=train_image, tile_size=(256, 256), tile_overlap=(48, 48), batch_size=1, )
Save predictions¶
In [7]:
Copied!
pred_folder = root / ("results_" + algo)
pred_folder.mkdir(exist_ok=True, parents=True)
final_data = np.concatenate(prediction)
tifffile.imwrite(pred_folder / "prediction.tiff", final_data)
pred_folder = root / ("results_" + algo) pred_folder.mkdir(exist_ok=True, parents=True) final_data = np.concatenate(prediction) tifffile.imwrite(pred_folder / "prediction.tiff", final_data)
Visualize the prediction¶
In [8]:
Copied!
n = 5
fig, ax = plt.subplots(3 * n, 2, figsize=(10, 3 * n * 5))
for i in range(n):
row = 3 * i
# channel 1
ax[row, 0].imshow(train_image[i, 0], cmap="gray")
ax[row, 0].set_title("Noisy - Channel 1")
ax[row, 1].imshow(prediction[i].squeeze()[0], cmap="gray")
ax[row, 1].set_title("Prediction - Channel 1")
# channel 2
ax[row + 1, 0].imshow(train_image[i, 1], cmap="gray")
ax[row + 1, 0].set_title("Noisy - Channel 2")
ax[row + 1, 1].imshow(prediction[i].squeeze()[1], cmap="gray")
ax[row + 1, 1].set_title("Prediction - Channel 2")
# channel 3
ax[row + 2, 0].imshow(train_image[i, 2], cmap="gray")
ax[row + 2, 0].set_title("Noisy - Channel 3")
ax[row + 2, 1].imshow(prediction[i].squeeze()[2], cmap="gray")
ax[row + 2, 1].set_title("Prediction - Channel 3")
n = 5 fig, ax = plt.subplots(3 * n, 2, figsize=(10, 3 * n * 5)) for i in range(n): row = 3 * i # channel 1 ax[row, 0].imshow(train_image[i, 0], cmap="gray") ax[row, 0].set_title("Noisy - Channel 1") ax[row, 1].imshow(prediction[i].squeeze()[0], cmap="gray") ax[row, 1].set_title("Prediction - Channel 1") # channel 2 ax[row + 1, 0].imshow(train_image[i, 1], cmap="gray") ax[row + 1, 0].set_title("Noisy - Channel 2") ax[row + 1, 1].imshow(prediction[i].squeeze()[1], cmap="gray") ax[row + 1, 1].set_title("Prediction - Channel 2") # channel 3 ax[row + 2, 0].imshow(train_image[i, 2], cmap="gray") ax[row + 2, 0].set_title("Noisy - Channel 3") ax[row + 2, 1].imshow(prediction[i].squeeze()[2], cmap="gray") ax[row + 2, 1].set_title("Prediction - Channel 3")
Cover¶
In [9]:
Copied!
# create a cover image
im_idx = 8
cv_image_noisy = train_image[im_idx]
cv_image_pred = prediction[im_idx].squeeze()
# create image
cover = np.zeros((3, 256, 256))
(_, height, width) = cv_image_noisy.shape
assert height > 256
assert width > 256
# get min and max and reshape them so that they can be broadcasted with the images
noise_min = np.array(np.min(cv_image_noisy, axis=(1, 2)))[
(..., *[np.newaxis] * (cv_image_noisy.ndim - 1))
]
noise_max = np.array(np.max(cv_image_noisy, axis=(1, 2)))[
(..., *[np.newaxis] * (cv_image_noisy.ndim - 1))
]
pred_min = np.array(np.min(cv_image_pred, axis=(1, 2)))[
(..., *[np.newaxis] * (cv_image_pred.ndim - 1))
]
pred_max = np.array(np.max(cv_image_pred, axis=(1, 2)))[
(..., *[np.newaxis] * (cv_image_pred.ndim - 1))
]
# normalize train and prediction per channel
norm_noise = (cv_image_noisy - noise_min) / (noise_max - noise_min)
norm_pred = (cv_image_pred - pred_min) / (pred_max - pred_min)
# fill in halves
cover[:, :, : 256 // 2] = norm_noise[
:,
height // 2 - 256 // 2 : height // 2 + 256 // 2,
width // 2 - 256 // 2 : width // 2,
]
cover[:, :, 256 // 2 :] = norm_pred[
:,
height // 2 - 256 // 2 : height // 2 + 256 // 2,
width // 2 : width // 2 + 256 // 2,
]
# move C axis at the end
cover = np.moveaxis(cover, 0, -1)
# plot the single image
plt.imshow(cover)
# save the image
plt.imsave("W2S_N2V.jpeg", cover)
# create a cover image im_idx = 8 cv_image_noisy = train_image[im_idx] cv_image_pred = prediction[im_idx].squeeze() # create image cover = np.zeros((3, 256, 256)) (_, height, width) = cv_image_noisy.shape assert height > 256 assert width > 256 # get min and max and reshape them so that they can be broadcasted with the images noise_min = np.array(np.min(cv_image_noisy, axis=(1, 2)))[ (..., *[np.newaxis] * (cv_image_noisy.ndim - 1)) ] noise_max = np.array(np.max(cv_image_noisy, axis=(1, 2)))[ (..., *[np.newaxis] * (cv_image_noisy.ndim - 1)) ] pred_min = np.array(np.min(cv_image_pred, axis=(1, 2)))[ (..., *[np.newaxis] * (cv_image_pred.ndim - 1)) ] pred_max = np.array(np.max(cv_image_pred, axis=(1, 2)))[ (..., *[np.newaxis] * (cv_image_pred.ndim - 1)) ] # normalize train and prediction per channel norm_noise = (cv_image_noisy - noise_min) / (noise_max - noise_min) norm_pred = (cv_image_pred - pred_min) / (pred_max - pred_min) # fill in halves cover[:, :, : 256 // 2] = norm_noise[ :, height // 2 - 256 // 2 : height // 2 + 256 // 2, width // 2 - 256 // 2 : width // 2, ] cover[:, :, 256 // 2 :] = norm_pred[ :, height // 2 - 256 // 2 : height // 2 + 256 // 2, width // 2 : width // 2 + 256 // 2, ] # move C axis at the end cover = np.moveaxis(cover, 0, -1) # plot the single image plt.imshow(cover) # save the image plt.imsave("W2S_N2V.jpeg", cover)